Compreendendo o papel da mobilidade interprovincial humana na disseminação da COVID-19: um modelo metapopulacional estocástico com taxas de contacto dependentes do tempo
DOI:
https://doi.org/10.14295/vetor.v35i1.18479Palavras-chave:
Pandemia de COVID-19, Mobilidade interprovincial, Modelo metapopulacional estocástico, Modelo de radiação, Taxas de contacto dependentes do tempoResumo
A modelação epidemiológica que incorpora a mobilidade interprovincial e as taxas de contacto variáveis no tempo é essencial para elucidar a dinâmica de transmissão da COVID-19. A migração de indivíduos entre províncias e as flutuações temporais nas taxas de contacto moldadas pelas directivas de distanciamento social são consideradas os factores cruciais que influenciam a propagação da doença. Para investigar estas variáveis, construímos um modelo metapopulacional estocástico composto por 11 subpopulações, cada uma delineada por um submodelo SEIAHRD compartimental, para articular a transmissão da COVID-19 no contexto de Moçambique. A mobilidade interprovincial foi quantificada utilizando uma matriz origem-destino derivada do modelo de radiação. A interação entre mobilidade humana e variabilidade nas taxas de contato foi avaliada ao testar três cenários distintos: modelo sem mobilidade; modelo com mobilidade e modelo com mobilidade e isolamento da Cidade de Maputo. O modelo que incorpora taxas de contato dependentes do tempo demonstrou desempenho superior em todos os cenários do modelo. Estes resultados sublinham a importância da mobilidade inter-regional na definição da dinâmica epidémica e sublinham o efeito das intervenções governamentais nas flutuações nas taxas de contacto. Esta investigação sublinha a necessidade de restrições estratégicas de mobilidade e medidas adaptativas nos futuros quadros de controlo epidémico para melhorar a eficácia da resposta e mitigar as repercussões sociais e de saúde.
Downloads
Referências
S. A. Iyaniwura, N. Ringa, P. A. Adu, S. Mak, N. Z. Janjua, M. A. Irvine, and M. Otterstatter, “Understanding the impact of mobility on COVID-19 spread: A hybrid gravity-metapopulation model of COVID-19,” PLOS Computational Biology, vol. 19, no. 5, p. e1011123, 2023. Available at: https://doi.org/10.1371/journal.pcbi.1011123
M. Chinazzi, J. T. Davis, M. Ajelli, C. Gioannini, M. Litvinova, S. Merler, A. P. y Piontti, K. Mu, L. Rossi, K. Sun, C. Viboud, X. Xiong, H. Yu, M. E. Halloran, I. M. Longini, and A. Vespignani, “The effect of travel restrictions on the spread of the 2019 novel coronavirus (COVID-19) outbreak,” Science, vol. 368, no. 6489, pp. 395–400, 2020. Available at: https://doi.org/10.1126/science.aba9757
M. Zhang, S. Wang, T. Hu, X. Fu, X. Wang, Y. Hu, B. Halloran, Z. Li, Y. Cui, H. Liu, Z. Liu, and S. Bao, “Human mobility and COVID-19 transmission: a systematic review and future directions,” Annals of GIS, vol. 28, no. 4, pp. 501–514, 2022. Available at: https://doi.org/10.1080/19475683.2022.2041725
Y. Wei, J. Wang, W. Song, C. Xiu, L. Ma, and T. Pei, “Spread of COVID-19 in China: analysis from a city-based epidemic and mobility model,” Cities, vol. 110, p. 103010, 2021. Available at: https://doi.org/10.1016/j.cities.2020.103010
G. S. Costa, W. Cota, and S. C. Ferreira, “Outbreak diversity in epidemic waves propagating through distinct geographical scales,” Physical Review Research, vol. 2, no. 4, p. 043306, 2020. Available at: https://doi.org/10.1103/PhysRevResearch.2.043306
A. Arenas, W. Cota, J. Gómez-Gardeñes, S. Gómez, C. Granell, J. T. Matamalas, D. Soriano-Paños, and B. Steinegger, “Modeling the spatiotemporal epidemic spreading of COVID-19 and the impact of mobility and social distancing interventions,” Physical Review X, vol. 10, no. 4, p. 041055, 2020. Available at: https://doi.org/10.1103/PhysRevX.10.041055
R. K. Eisfeld and T. Just, “Urban density versus regional dispersion: On the risks in high-density conurbations in Germany during COVID-19,” Sustainable Cities and Society, vol. 108, p. 105503, 2024. Available at: https://doi.org/10.1016/j.scs.2024.105503
G. Pullano, L. G. Alvarez-Zuzek, V. Colizza, and S. Bansal, “Characterizing US spatial connectivity: implications for geographical disease dynamics and metapopulation modeling,” medRxiv, pp. 2023–11, 2023. Available at: https://doi.org/10.1101/2023.11.22.23298916
P. S. Peixoto, D. Marcondes, C. Peixoto, and S. M. Oliva, “Modeling future spread of infections via mobile geolocation data and population dynamics. An application to COVID-19 in Brazil,” PloS One, vol. 15, no. 7, p. e0235732, 2020. Available at: https://doi.org/10.1371/journal.pone.0235732
M. H. H. S. Uiterkamp, M. Gösgens, H. Heesterbeek, R. van der Hofstad, and N. Litvak, “The role of inter-regional mobility in forecasting SARS-CoV-2 transmission,” Journal of the Royal Society Interface, vol. 19, no. 193, p. 20220486, 2022. Available at: https://doi.org/10.1098/rsif.2022.0486
E. Prestige, P. Coletti, J. Backer, N. G. Davies, W. J. Edmunds, and C. I. Jarvis, “Estimating social contact rates for the COVID-19 pandemic using Google mobility and pre-pandemic contact surveys,” medRxiv, pp. 2023–12, 2023. Available at: https://doi.org/10.1101/2023.12.19.23300209
S. Flaxman, S. Mishra, A. Gandy, H. J. T. Unwin, T. A. Mellan, H. Coupland, C. Whittaker, H. Zhu, T. Berah, J. W. Eaton, M. Monod, P. N. Perez-Guzman, N. Schmit, L. Cilloni, K. E. C. Ainslie, M. Baguelin, A. Boonyasiri, O. Boyd, L. Cattarino, L. V. Cooper, Z. Cucunubá, G. Cuomo-Dannenburg, A. Dighe, B. Djaafara, I. Dorigatti, S. L. van Elsland, R. G. FitzJohn, K. A. M. Gaythorpe, L. Geidelberg, N. C. Grassly, W. D. Green, T. Hallett, A. Hamlet, W. Hinsley, B. Jeffrey, E. Knock, D. J. Laydon, G. Nedjati-Gilani, P. Nouvellet, K. V. Parag, I. Siveroni, H. A. Thompson, R. Verity, E. Volz, C. E. Walters, H. Wang, Y. Wang, O. J. Watson, P. Winskill, X. Xi, P. G. T. Walker, A. C. Ghani, C. A. Donnelly, S. Riley, M. A. C. Vollmer, N. M. Ferguson, L. C. Okell, and S. Bhatt, “Estimating the effects of non-pharmaceutical interventions on COVID-19 in Europe,” Nature, vol. 584, no. 7820, pp. 257–261, 2020. Available at: https://doi.org/10.1038/s41586-020-2405-7
G. Pullano, E. Valdano, N. Scarpa, S. Rubrichi, and V. Colizza, “Population mobility reductions during COVID-19 epidemic in France under lockdown,” MedRxiv, vol. 29, p. 2020, 2020. Available at: https://www.epicx-lab.com/uploads/9/6/9/4/9694133/inserm-covid-19_report_mobility_fr_lockdown-20200511.pdf
J. Arino and P. van den Driessche, “A multi-city epidemic model,” Mathematical Population Studies, vol. 10, no. 3, pp. 175–193, 2003. Available at: https://doi.org/10.1080/08898480306720
T. Dhirasakdanon, H. R. Thieme, and P. Van Den Driessche, “A sharp threshold for disease persistence in host metapopulations,” Journal of Biological Dynamics, vol. 1, no. 4, pp. 363–378, 2007. Available at: https://doi.org/10.1080/17513750701605465
A. Wesolowski, C. O. Buckee, K. Engø-Monsen, and C. J. E. Metcalf, “Connecting mobility to infectious diseases: the promise and limits of mobile phone data,” The Journal of Infectious Diseases, vol. 214, no. suppl_4, pp. S414–S420, 2016. Available at: https://doi.org/10.1093/infdis/jiw273
Z. Rapti, J. Cuevas-Maraver, E. Kontou, S. Liu, Y. Drossinos, P. G. Kevrekidis, M. Barmann, Q.-Y. Chen, and G. A. Kevrekidis, “The role of mobility in the dynamics of the COVID-19 epidemic in Andalusia,” Bulletin of Mathematical Biology, vol. 85, no. 6, p. 54, 2023. Available at: https://doi.org/10.1007/s11538-023-01152-5
MISAU. (2020) Coronovirus. Covid-19 boletins diário. Available at: https://www.misau.gov.mz/index.php/covid-19-boletins-diarios
Z. Liu, P. Magal, O. Seydi, and G. Webb, “A COVID-19 epidemic model with latency period,” Infectious Disease Modelling, vol. 5, pp. 323–337, 2020. Available at: https://doi.org/10.1016/j.idm.2020.03.003
V. A. Karatayev, M. Anand, and C. T. Bauch, “Local lockdowns outperform global lockdown on the far side of the COVID-19 epidemic curve,” Proceedings of the National Academy of Sciences, vol. 117, no. 39, pp. 24 575–24 580, 2020. Available at: https://doi.org/10.1073/pnas.2014385117
S. He, S. Tang, and L. Rong, “A discrete stochastic model of the COVID-19 outbreak: Forecast and control,” Mathematical Biosciences and Engineering, vol. 17, no. 4, pp. 2792–2804, 2020. Available at: http://doi.org/10.3934/mbe.2020153
A. P. Masucci, J. Serras, A. Johansson, and M. Batty, “Gravity versus radiation models: On the importance of scale and heterogeneity in commuting flows,” Physical Review E – Statistical, Nonlinear, and Soft Matter Physics, vol. 88, no. 2, p. 022812, 2013. Available at: https://doi.org/10.1103/PhysRevE.88.022812
F. Simini, M. C. González, A. Maritan, and A.-L. Barabási, “A universal model for mobility and migration patterns,” Nature, vol. 484, no. 7392, pp. 96–100, 2012. Available at: https://doi.org/10.1038/nature10856
M. Tizzoni, P. Bajardi, A. Decuyper, G. Kon Kam King, C. M. Schneider, V. Blondel, Z. Smoreda, M. C. González, and V. Colizza, “On the use of human mobility proxies for modeling epidemics,” PLoS Computational Biology, vol. 10, no. 7, p. e1003716, 2014. Available at: https://doi.org/10.1371/journal.pcbi.1003716
P. C. Ventura, A. Aleta, F. A. Rodrigues, and Y. Moreno, “Modeling the effects of social distancing on the large-scale spreading of diseases,” Epidemics, vol. 38, p. 100544, 2022. Available at: https://doi.org/10.1016/j.epidem.2022.100544
L. Pappalardo, F. Simini, G. Barlacchi, and R. Pellungrini, “scikit-mobility: A python library for the analysis, generation and risk assessment of mobility data,” arXiv, 2019. Available at: https://doi.org/10.48550/arXiv.1907.07062
S. A. Pedro, F. T. Ndjomatchoua, P. Jentsch, J. M. Tchuenche, M. Anand, and C. T. Bauch, “Conditions for a second wave of COVID-19 due to interactions between disease dynamics and social processes,” Frontiers in Physics, vol. 8, p. 574514, 2020. Available at: https://doi.org/10.3389/fphy.2020.574514
A. Cassy, T. Marrufo, and S. Chicumbe, “COVID-19 em Moçambique, relatório do 1o ano, 2020-2021,” Instituto Nacional de Saúde, Marracuene, Mozambique, Tech. Rep. 1, 2021. Available at: https://ons.nilzachipe.com/wp-content/uploads/2024/03/ONS-Relatorio-do-1o-Ano-da-COVID-19-Mocambique.pdf
A. W. Byrne, D. McEvoy, A. B. Collins, K. Hunt, M. Casey, A. Barber, F. Butler, J. Griffin, E. A. Lane, C. McAloon et al., “Inferred duration of infectious period of SARS-CoV-2: rapid scoping review and analysis of available evidence for asymptomatic and symptomatic COVID-19 cases,” BMJ Open, vol. 10, no. 8, p. e039856, 2020. Available at: https://doi.org/10.1136/bmjopen-2020-039856
T. Akiba, S. Sano, T. Yanase, T. Ohta, and M. Koyama, “Optuna: A next-generation hyperparameter optimization framework,” in Proceedings of the 25th ACM SIGKDD International Conference on Knowledge Discovery & Data Mining, 2019, pp. 2623–2631.
A. Saltelli, Global Sensitivity Analysis: the Primer, 1st ed. West sussexPO19 8SQ, England: John Wiley & Sons, 2008. Available at: https://www.andreasaltelli.eu/file/repository/A_Saltelli_Marco_Ratto_Terry_Andres_Francesca_Campolongo_Jessica_Cariboni_Debora_Gatelli_Michaela_Saisana_Stefano_Tarantola_Global_Sensitivity_Analysis_The_Primer_Wiley_Interscience_2008_.pdf
J. Herman and W. Usher, “Salib: An open-source python library for sensitivity analysis,” Journal of Open Source Software, vol. 2, no. 9, p. 97, 2017. Available at: https://doi.org/10.21105/joss.00097
F. Gugole, L. E. Coffeng, W. Edeling, B. Sanderse, S. J. De Vlas, and D. Crommelin, “Uncertainty quantification and sensitivity analysis of COVID-19 exit strategies in an individual-based transmission model,” PLoS Computational Biology, vol. 17, no. 9, p. e1009355, 2021. Available at: https://doi.org/10.1371/journal.pcbi.1009355
D. A. Buch, J. E. Johndrow, and D. B. Dunson, “Explaining transmission rate variations and forecasting epidemic spread in multiple regions with a semiparametric mixed effects SIR model,” Biometrics, vol. 79, no. 4, pp. 2987–2997, 2023. Available at: https://doi.org/10.1111/biom.13901
M. U. G. Kraemer, C.-H. Yang, B. Gutierrez, C.-H. Wu, B. Klein, D. M. Pigott, L. du Plessis, N. R. Faria, R. Li, W. P. Hanage, J. S. Brownstein, M. Layan, A. Vespignani, H. Tian, C. Dye, O. G. Pybus, and S. V. Scarpino, “The effect of human mobility and control measures on the COVID-19 epidemic in China,” Science, vol. 368, no. 6490, pp. 493–497, 2020. Available at: https://doi.org/10.1126/science.abb4218
F. Pedregosa, G. Varoquaux, A. Gramfort, V. Michel, B. Thirion, O. Grisel, M. Blondel, P. Prettenhofer, R. Weiss, V. Dubourg, J. Vanderplas, A. Passos, D. Cournapeau, M. Brucher, M. Perrot, and E. Duchesnay, “Scikit-learn: Machine learning in python,” The Journal of Machine Learning Research, vol. 12, pp. 2825–2830, 2011. Available at: https://www.jmlr.org/papers/volume12/pedregosa11a/pedregosa11a.pdf?ref=https:/
P. Joaquim, D. Takahashi, and S. Pedro, “The role of interprovincial mobility in the dynamics of COVID-19 epidemic in mozambique: Insights from a stochastic metapopulation model,” in Anais do Encontro Nacional de Modelagem Computacional e Encontro de Ciência e Tecnologia de Materiais, XXVII Encontro Nacional de Modelagem Computacional e XV Encontro de Ciência e Tecnologia dos Materiais. Ilhéus, Brasil: Even3, 2024. Available at: https://www.even3.com.br/anais/enmc2024/914710-the-role-of-interprovincial-mobility-in-the-dynamics-of-covid-19-epidemic-in-mozambique--insights-from-a-stochast
N. C. Grassly and C. Fraser, “Mathematical models of infectious disease transmission,” Nature Reviews Microbiology, vol. 6, no. 6, pp. 477–487, 2008. Available at: https://doi.org/10.1038/nrmicro1845
J. M. Brauner, S. Mindermann, M. Sharma, D. Johnston, J. Salvatier, T. Gavenčiak, A. B. Stephenson, G. Leech, G. Altman, V. Mikulik, A. J. Norman, J. T. Monrad, T. Besiroglu, H. Ge, M. A. Hartwick, Y. W. Teh, L. Chindelevitch, Y. Gal, and J. Kulveit, “Inferring the effectiveness of government interventions against COVID-19,” Science, vol. 371, no. 6531, p. eabd9338, 2021. Available at: https://doi.org/10.1126/science.abd9338
F. Arroyo-Marioli, F. Bullano, S. Kucinskas, and C. Rondón-Moreno, “Tracking R of COVID-19: A new real-time estimation using the Kalman filter,” PloS One, vol. 16, no. 1, p. e0244474, 2021. Available at: https://doi.org/10.1371/journal.pone.0244474
R. A. Neher, R. Dyrdak, V. Druelle, E. B. Hodcroft, and J. Albert, “Impact of seasonal forcing on a potential SARS-CoV-2 pandemic,” medRxiv, pp. 2020–02, 2020. Available at: https://doi.org/10.1101/2020.02.13.20022806
A. H. Khan, Challenges in Modeling of an Outbreak’s Prediction, Forecasting and Decision Making for Policy Makers. Singapore: Springer Singapore, 2021, pp. 377–408. Available at: https://doi.org/10.1007/978-981-16-2450-6_17
K.-F. Chen, T.-W. Feng, C.-C. Wu, I. Yunusa, S.-H. Liu, C.-F. Yeh, S.-T. Han, C.-Y. Mao, D. Harika, R. Rothman, and A. Pekosz, “Diagnostic accuracy of clinical signs and symptoms of COVID-19: A systematic review and meta-analysis to investigate the different estimates in a different stage of the pandemic outbreak,” Journal of Global Health, vol. 13, 2023. Available at: https://doi.org/10.7189/jogh.13.06026
L. Ma, Z. Qiu, P. Van Mieghem, and M. Kitsak, “Reporting delays: A widely neglected impact factor in COVID-19 forecasts,” PNAS Nexus, vol. 3, no. 6, 2024. Available at: https://doi.org/10.1093/pnasnexus/pgae204
K. Gallagher, R. Creswell, D. Gavaghan, and B. Lambert, “Identification and attribution of weekly periodic biases in epidemiological time series data,” medRxiv, pp. 2023–06, 2023. Available at: https://doi.org/10.1101/2023.06.13.23290903
S. Engblom, R. Eriksson, and S. Widgren, “Bayesian epidemiological modeling over high-resolution network data,” Epidemics, vol. 32, p. 100399, 2020. Available at: https://doi.org/10.1016/j.epidem.2020.100399
K. Prem, A. R. Cook, and M. Jit, “Projecting social contact matrices in 152 countries using contact surveys and demographic data,” PLoS Computational Biology, vol. 13, no. 9, p. e1005697, 2017. Available at: https://doi.org/10.1371/journal.pcbi.1005697
A. Machens, F. Gesualdo, C. Rizzo, A. E. Tozzi, A. Barrat, and C. Cattuto, “An infectious disease model on empirical networks of human contact: bridging the gap between dynamic network data and contact matrices,” BMC Infectious Diseases, vol. 13, pp. 1–15, 2013. Available at: https://doi.org/10.1186/1471-2334-13-185
Y. Li, T. Hu, X. Gai, Y. Zhang, and X. Zhou, “Transmission dynamics, heterogeneity and controllability of SARS-CoV-2: a rural–urban comparison,” International Journal of Environmental Research and Public Health, vol. 18, no. 10, p. 5221, 2021. Available at: https://doi.org/10.3390/ijerph18105221
J. Chen, P. Bhattacharya, S. Hoops, D. Machi, A. Adiga, H. Mortveit, S. Venkatramanan, B. Lewis, and M. Marathe, “Role of heterogeneity: National scale data-driven agent-based modeling for the US COVID-19 scenario modeling hub,” Epidemics, vol. 48, p. 100779, 2024. Available at: https://doi.org/10.1016/j.epidem.2024.100779
A. L. Bertozzi, E. Franco, G. Mohler, M. B. Short, and D. Sledge, “The challenges of modeling and forecasting the spread of COVID-19,” Proceedings of the National Academy of Sciences, vol. 117, no. 29, pp. 16 732–16 738, 2020. Available at: https://doi.org/10.1073/pnas.2006520117
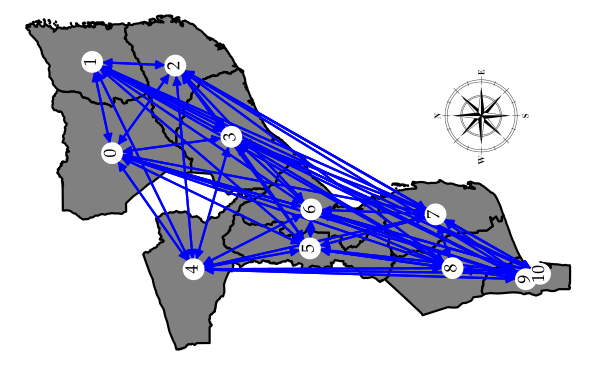