Identificação do Movimento Linear da Série Temporal do Volume Mensal de Transações da Criptomoeda Ethereum por meio do Modelo SARIMA
DOI:
https://doi.org/10.14295/vetor.v33i1.15162Palavras-chave:
Série Temporal, Ethereum, SARIMAResumo
Esse trabalho apresenta a modelagem do volume mensal de transações da criptmoeda Ethereum por meio da metodologia de Box-Jenkins, envolvendo as etapas: análise exploratória, identificação, estimação e validação, algumas das quais executadas com a utilização de diferentes técnicas. O modelo encontrado pela modelagem SARIMA (Modelo autoregressivo integrado de médias móveis sazonal) conseguiu descrever o comportamento linear dos dados de forma satisfatória, mas não foi suficiente para descrever o comportamento da série, composta por movimento linear e não linear, sendo melhor representada por um modelo híbrido.Downloads
Referências
L. H. A. Müller, “Caminhos e sentidos da informação no mercado de ações,” International Review of Economics Finance, vol. 4, no. 6, pp. 133–164, 2005.
P. A. Morettin, Econometria financeira: um curso em séries temporais financeiras, 3a ed. São Paulo: Blucher, 2011.
J. D. V. Henao, V. M. R. Mejia, e C. J. F. Cardona, “Electricity demand forecasting using a SARIMA-multiplicative single neuron hybrid model,” DYNA, vol. 80, pp. 4–8, 2013. Disponível em: http://ref.scielo.org/b84jzw
C. Sigauke, “Forecasting medium-term electricity demand in a South African electric power supply system,” Journal of Energy in Southern Africa, vol. 28, pp. 54–67, 2017. Disponível em: https://doi.org/10.17159/2413-3051/2017/v28i4a2428
Y. Yang, Y. Chen, Y. Wang, C. Li, e L. Li, “Modelling a combined method based on ANFIS and neural network improved by DE algorithm: A case study for short-term electricity demand forecasting,” Applied Soft Computing, vol. 49, pp. 663–675, 2016. Disponível em: https://www.sciencedirect.com/science/article/pii/S1568494616303891
G. E. P. Box e G. M. Jenkins, Time series analysis : forecasting and control. San Francisco: Holden-Dayr, 1976.
P. A. Morettin e C. M. de Castro Toloi, Análise de séries temporais, 3a ed. São Paulo: Blucher, 2018.
V. L. D. de Mattos, L. R. Nakamura, A. C. Konrath, e A. C. Bornia, “Modeling the commercial electricity demand in Santa Catarina, using the Box-Jenkins methodology,” International Journal of Development Research, vol. 11, 2021, artigo no. 22265. Disponível em: https://doi.org/10.37118/ijdr.22265.06.2021
T. P. Pereira, V. L. D. de Mattos, A. C. Konrath, A. Bornia, L. R. Nakamura, e V. Vargas, “Estudo sobre testes de sazonalidade no volume mensal de transações da criptmoeda Ethereum,” em Anais da 20a Mostra de Produção Universitária (MPU – FURG). Rio Grande, Brasil: Universidade Federal do Rio Grande, 2021.
P. S. Thomaz e V. L. D. de Mattos, “Predicting the GFCF of the brazilian construction industry: a comparison between holt winters’ and SARIMA models,” Revista Gestão Industrial, vol. 15, no. 1, pp. 180–196, 2019. Disponível em: https://doi.org/10.3895/gi.v15n1.8590
I. Tahyudin, R. Wahyudi, e H. Nambo, “SARIMA-LSTM combination for COVID-19 case modeling,” IIUM Engineering Journal, vol. 23, no. 2, p. 171–182, 2022. Disponível em: https://doi.org/10.31436/iiumej.v23i2.2134
(2022) Coinmarketcap. Disponível em: https://coinmarketcap.com/
G. C. Chow, “Tests of equality between sets of coefficients in two linear regressions,” Econometrica, vol. 28, no. 3, pp. 591–605, 1960. Disponível em: https://doi.org/10.2307/1910133
D. A. Dickey e W. A. Fuller, “Likelihood ratio statistics for autoregressive time series with a unit root,” Econometrica, vol. 49, no. 4, pp. 1057–1072, 1981. Disponível em: https://doi.org/10.2307/1912517
D. Kwiatkowski, P. C. Phillips, P. Schmidt, e Y. Shin, “Testing the null hypothesis of stationarity against the alternative of a unit root: How sure are we that economic time series have a unit root?” Journal of Econometrics, vol. 54, no. 1, pp. 159–178, 1992. Disponível em: https://doi.org/10.1016/0304-4076(92)90104-Y
S. Hylleberg, R. Engle, C. Granger, e B. Yoo, “Seasonal integration and cointegration,” Journal of Econometrics, vol. 44, no. 1, pp. 215–238, 1990. Disponível em: https://doi.org/10.1016/0304-4076(90)90080-D
D. R. Osborn, A. P. L. Chui, J. P. Smith, e C. R. Birchenhall, “Seasonality and the order of integration for consumption,” Oxford Bulletin of Economics and Statistics, vol. 50, no. 4, pp. 361–377, 1988. Disponível em: https://doi.org/10.1111/j.1468-0084.1988.mp50004002.x
G. Schwarz, “Estimating the dimension of a model,” The Annals of Statistics, vol. 6, no. 2, pp. 461–464, 1978. Disponível em: http://www.jstor.org/stable/2958889
C. M. Jarque e A. K. Bera, “Efficient tests for normality, homoscedasticity and serial independence of regression residuals,” Economics Letters, vol. 6, no. 3, pp. 255–259, 1980. Disponível em: https://doi.org/10.1016/0165-1765(80)90024-5
R. F. Engle, “Autoregressive conditional heteroscedasticity with estimates of the variance of United Kingdom inflation,” Econometrica, vol. 50, no. 4, pp. 987–1007, 1982. Disponível em: https://doi.org/10.2307/1912773
R Core Team. (2023) R: a language and environment for statistical computing.viena, austria: R core team. Disponível em: https://www.r-project.org/
R. Hyndman, G. Athanasopoulos, C. Bergmeir, G. Caceres, L. Chhay, K. Kuroptev, M. O’Hara-Wild, F. Petropoulos, S. Razbash, E. Wang, F. Yasmeen, F. Garza, D. Girolimetto, R. Ihaka, D. Reid, D. Shaub, Y. Tang, X. Wang, e Z. Zhou. (2023) forecast: Forecasting functions for time series and linear models. Disponível em: https://pkg.robjhyndman.com/forecast/,https://github.com/robjhyndman/forecast
A. Trapletti, K. Hornik, e B. LeBaron. (2023) tseries: Time series analysis and computational finance. Disponível em: https://CRAN.R-project.org/package=tseries
D. Meyer, E. Dimitriadou, K. Hornik, A. Weingessel, F. Leisch, C.-C. Chang, e C.-C. Lin. (2023) e1071: Misc functions of the department of statistics, probability theory group. Disponível em: https://CRAN.R-project.org/package=e1071
G. N. Boshnakov. (2018) Fints: Companion to Tsay analysis of financial time séries. Disponível em: https://r-forge.r-project.org/projects/fints/
H. Wickham, W. Chang, T. L. Pedersen, K. Takahashi, C. Wilke, K. Woo, H. Yutani, e D. Dunnington. (2023) ggplot2: Create elegant data visualisations using the grammar of graphics. Disponível em: https://ggplot2.tidyverse.org,https://github.com/tidyverse/ggplot2
The Gretl Team. (2018) Gretl: GNU regression, econometrics and time-séries library. Disponível em: https://gretl.sourceforge.net/pt.html
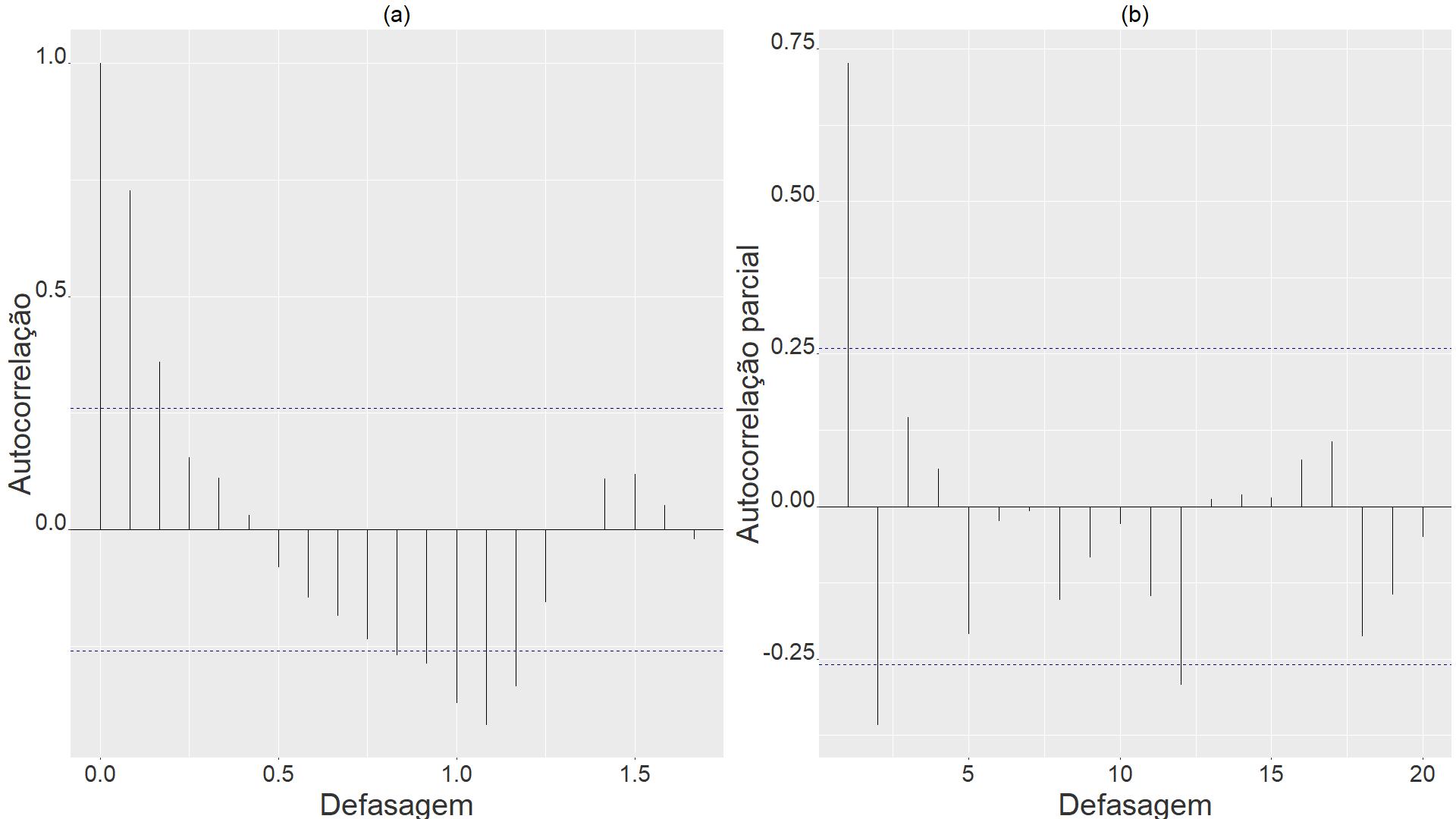